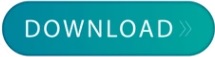

In summary, we argue that the next generation of AI must embrace “dark” humanlike common sense for solving novel tasks. Uses a combination of raytracing and multiple hill shading methods to produce 2D and 3D data visualizations and maps.
Full map overlay of 3d maze screensaver how to#
We demonstrate the power of this perspective to develop cognitive AI systems with humanlike common sense by showing how to observe and apply FPICU with little training data to solve a wide range of challenging tasks, including tool use, planning, utility inference, and social learning. Just as our universe cannot be understood by merely studying observable matter, we argue that vision cannot be understood without studying FPICU. Screensaver has been tested and declared for Windows Vista. Chapter 25 describes how to generate a 3D maze from a text file specification. Plasma Colors is OpenGL screensaver with smooth plasma animation and transition.
Full map overlay of 3d maze screensaver free#
Eye-catching abstract free screensaver with plasma effects. Dots randomly move, forming connections between each other. Imagine that you are flying through space with dots. Now display width/height ratio is fixed to 4:3. 76 An Almost Full-Screen (AFS) Worm An Undecorated Full-Screen (UFS) Worm. Very attractive abstract free 3D screensaver with special effects. Version 2.0.0: Smother screensaver starting has been released. We therefore coin them the “dark matter” of vision. Number of various 3D mazes 3D Maze ScreenSaver can generate is practically infinite. They are invisible in terms of pixels but nevertheless drive the creation, maintenance, and development of visual scenes. When taken as a unified concept, FPICU is concerned with the questions of “why” and “how,” beyond the dominant “what” and “where” framework for understanding vision. We identify functionality, physics, intent, causality, and utility (FPICU) as the five core domains of cognitive AI with humanlike common sense. We illustrate the potential power of this new paradigm by reviewing models of common sense that synthesize recent breakthroughs in both machine and human vision. Specifically, we propose a “small data for big tasks” paradigm, wherein a single artificial intelligence (AI) system is challenged to develop “common sense,” enabling it to solve a wide range of tasks with little training data. In this paper, we call for a shift that flips this paradigm upside down. Recent progress in deep learning is essentially based on a “big data for small tasks” paradigm, under which massive amounts of data are used to train a classifier for a single narrow task.
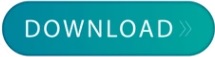